The Cost of Building AI: Understanding AI Cost Analysis
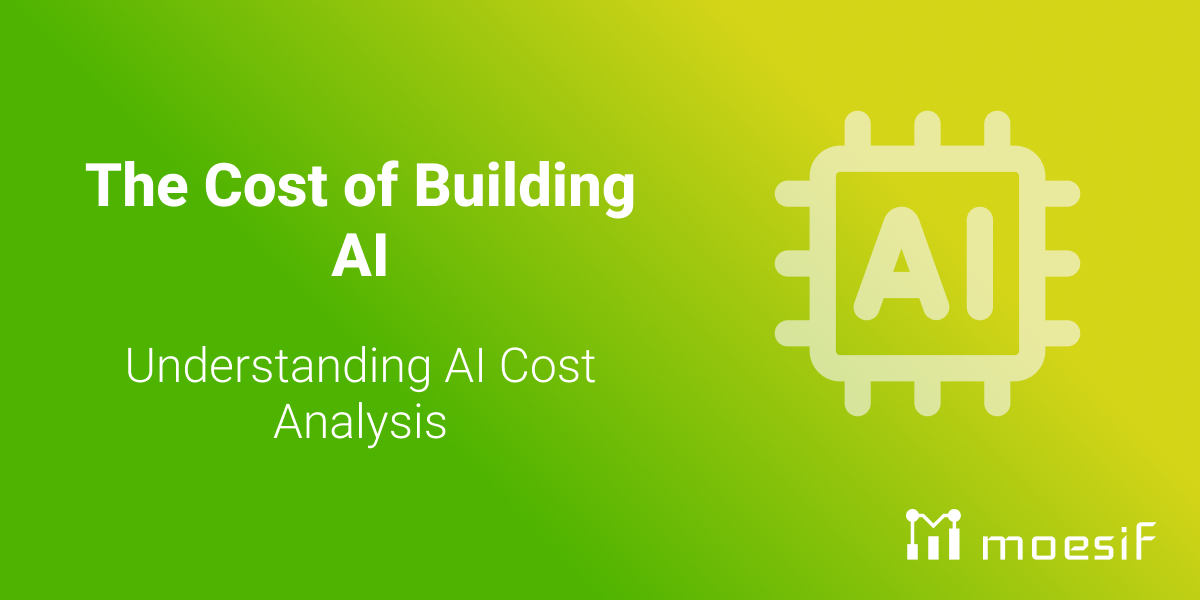
Technology generally comes with a cost. At scale, this cost can become exponential and quickly be challenging to manage. Artificial intelligence (AI) has come to the forefront of almost every vertical, finding ways to somehow improve the lives of nearly everyone on the planet. Everyone and every company is either using AI or building it.
However, as with any technology, building and deploying AI systems often comes with significant costs. The underlying hardware and expertise that make cutting-edge AI platforms possible is quite pricey. With so much at stake, understanding the intricacies of AI cost analysis is crucial for organizations to make informed decisions and ensure a positive return on investment (ROI) for their AI initiatives. This blog will look at the key factors contributing to the cost of building AI, explore cost-saving strategies, and outline pricing models for different AI solutions. We’ll also discuss essential steps for successful AI implementation and how tools like Moesif can assist in analyzing AI costs. Let’s begin by understanding the costs associated with AI.
Understanding AI Costs
The cost of building and implementing AI systems can vary significantly. Are you leveraging an existing AI platform to power your application’s AI functionality or building something from the ground up? Are you using pre-trained models or training them independently with a large data set? These factors lead to a better understanding of the initial costs of building AI applications and the long-term support costs. Let’s dig further into the critical elements that influence these costs:
Factors Affecting AI Cost
Some factors that affect AI cost are generic to all technology initiatives. Others are hyper-specific to AI solutions. Here are a few factors to focus on when considering the costs of an AI project:
Type of AI Software or Solution
Depending on the type of AI solution you want to build and how you build it will have a massive impact on the cost. This factor encompasses some of the other factors below, such as expertise, since creating a custom solution will require more expertise than integrating with a pre-built solution. Overall, this likely has the most considerable impact on the cost of your project, especially in the beginning stages. The two routes most companies take include:
- Building a Custom AI Solution: Developing tailor-made AI solutions to address specific business needs typically involves higher costs due to extensive research, development, and customization. These solutions offer maximum flexibility and control but require significant investment in time and resources.
- Using a Pre-built AI Solution: Off-the-shelf AI solutions, like chatbots or sentiment analysis tools, often come with lower upfront costs. However, they may lack the customization options and scalability required for complex AI projects.
Project Complexity
This factor examines the scope and scale of your AI platform. Does it require a lot of data from different integrations or sources, or is the functionality limited? If the project needs to scale to massive volumes of users and data, this can also affect costs as operations ramp up. Here are a few particulars to focus on when project complexity is taken into consideration:
- Features and Integrations: The more features and integrations an AI system requires, the more complex and costly it becomes. Each additional functionality demands development effort, testing, and ongoing maintenance.
- Scalability: Scalability becomes a significant cost factor if the AI system needs to handle increasing workloads or expand to new areas. Building scalable AI infrastructure often involves investment in additional hardware, software, and cloud resources.
Expertise of Data Scientists and Developers
Unsurprisingly, AI and ML developers are among the most costly to hire in the current market. The more expertise they have, the higher the price tag. Do you need an entire team of AI experts? It’s time to open up the pocketbook. Here are a few factors to consider when looking at the cost impact of the team you need to build and maintain your AI application:
- Skill Level: Experienced data scientists and AI developers with specialized skills often require higher salaries. Their expertise is crucial for complex projects but can contribute to increased labor costs.
- Team Size: The size of the development team directly impacts project costs. Larger teams may accelerate development but also increase the amount spent on payroll.
Data Requirements
AI models require data, and generally, lots of it. Moving data around, getting it into the proper format, and all the infrastructure costs for these data pipelines can be expensive. Although data infrastructure costs have come down quite a bit, the increase in volume and velocity of data sometimes makes any savings a wash. Here are a few ways that the data side of AI can impact costs:
- Data Collection and Annotation: Acquiring and labeling large volumes of high-quality data for training AI models can be expensive, especially if manual annotation or specialized data sources are required.
- Data Storage and Management: Storing and managing massive datasets can incur significant costs, particularly if cloud storage or specialized data management tools are required.
Algorithm Accuracy and Fluency
Some models are more expensive to run than others. Some are more expensive to run, others to train, etc. Depending on the algorithms and models you require for your AI platform, cost will likely be affected. When it comes to algorithm accuracy and fluency, here are some ways this can influence cost:
- Model Training: Developing AI models with high accuracy and fluency often involves iterative training processes, consuming computational resources and time.
- Model Optimization: Fine-tuning AI models for optimal performance can require additional development effort, further impacting project costs.
Of course, factors beyond these affect cost, but this covers the big-ticket items you should consider when beginning your AI cost analysis. Your next question is how you can save money when considering these factors. That’s precisely what’s next on our agenda.
Cost Savings Strategies
While building AI systems can be expensive, organizations can look to various strategies to optimize costs. Many general cost-saving methods work well in AI projects, but there are also a few AI-specific cost-saving strategies to consider, such as using pre-trained models. Let’s look at four ways to reduce the costs of AI projects.
- Thorough Planning: As with all software projects, careful planning is essential to minimize unexpected expenses. Before starting development, define clear project goals, identify potential risks, and create a detailed budget.
- Minimum Viable Product (MVP): Another fundamental aspect of software cost savings is starting with a smaller, functional version of your AI solution (an MVP). This can help test its feasibility and gather feedback so you aren’t wasting money on development that doesn’t matter to users. This approach helps validate the concept before investing in full-scale development.
- Pre-trained AI Models: Whenever possible, utilize pre-trained AI models as a foundation for your project. This can significantly reduce development time and costs compared to building models from scratch. Many companies offer pre-trained models that are incredibly efficient for most use cases.
- Iterative Development: Embrace an iterative development process where you continuously refine and improve your AI solution based on user feedback and real-world data. This lets you identify and address issues early, avoiding costly rework later.
By understanding the factors influencing AI costs and implementing as many cost-saving strategies as possible, organizations can navigate the complexities of AI development and maximize their return on investment.
AI System Cost Factors
Understanding an AI system’s components and associated costs is critical for keeping projects fiscally efficient. Not considering the labor costs associated with designing and building AI systems, data and infrastructure are the most significant costs within an AI system. When budgeting for building and supporting an AI system, it’s essential to consider the costs associated with both data and infrastructure:
Data-Related Costs
AI is not possible without massive amounts of data. Unfortunately, not all data is AI-ready since many systems require data to be in specific formats and stored on specific platforms. This can get quite expensive to ramp up and maintain. Here are a few data-related costs that you should factor into the potential cost of your AI system:
- Data Collection and Annotation: Gathering the necessary data for training AI models can be a significant expense. This includes collecting raw data from various sources, cleaning it to remove errors and inconsistencies, and annotating it (labeling it for machine learning).
- Data Storage and Management: Storing and managing large datasets requires extensive infrastructure, which can incur costs for cloud storage, database management systems, and data security measures.
- Data Quality and Preprocessing: Ensuring high-quality data is crucial for training accurate AI models since models are only as good as the data they are trained on. This involves preprocessing data to remove noise, handling missing values, and standardizing formats, which can require specialized tools and expertise to implement.
- Historical Data Costs: Accessing and utilizing historical data for training AI models may involve licensing fees or subscriptions to data providers.
Infrastructure Costs
As Nvidia has learned, infrastructure costs for creating AI systems are high. These platforms require a massive amount of heavily-optimized computing power. While companies developing the tech for this are seeing record profits, those using the tech are seeing significant bills, whether from self-hosted servers or soaring cloud computing costs. Here are some areas to consider when assessing infrastructure costs:
- Hardware Costs: AI model training often requires powerful hardware, particularly graphics processing units (GPUs), which can be expensive to purchase and maintain. Specialized hardware like Tensor Processing Units (TPUs) may also be necessary for specific AI workloads.
- Software Costs: Developing and deploying AI systems require specialized software tools and frameworks, such as TensorFlow or PyTorch, which may have licensing or subscription costs.
- Cloud Infrastructure Costs: Many organizations leverage AWS, Microsoft Azure, or Google Cloud Platform for AI development and deployment in the cloud. These platforms offer scalable infrastructure and pay-as-you-go pricing models, but the costs can quickly increase depending on usage. Thankfully, if you understand where user demand is heading, its relatively easy to forecast spend on these platforms.
- IT Infrastructure Costs: If your AI system is deployed on-premises, you’ll need to factor in the costs of servers, storage, networking equipment, and other IT infrastructure components.
To accurately forecast the costs of your AI system, you must carefully consider these data and infrastructure cost factors. These are critical components in helping organizations develop a realistic budget for their AI initiatives, ensuring they have the resources necessary to build and deploy successful AI systems.
Understanding the Total Cost of Ownership of AI Systems
In addition to the factors mentioned earlier, understanding the cost breakdown of different components involved in building AI systems is crucial for effective budgeting and resource allocation. Often, you’ll hear a business talk about the Total Cost of Ownership, or TCO, of an application they are building or buying. Looking at TCO requires looking at every possible expense a system incurs when building, deploying, and maintaining it. When we are talking about building AI systems, we want to factor TCO into our cost analysis to ensure that revenue is generated and not lost by adding AI.
Let’s look further at the typical cost elements that should be factored into the TCO of an AI application:
Personnel Costs
These are the dollars you put out in terms of salaries, contractors, and consultants. Generally, these costs are highest as a platform is being developed versus when it is deployed and goes into support mode. The ebbs and flows of these costs should be calculated based on the application roadmap. For AI applications, here are the buckets of personnel to be aware of:
- Data Scientists and AI Engineers: These experts play a pivotal role in developing and fine-tuning AI models. Their salaries and associated benefits can constitute a significant portion of the budget, especially for complex projects.
- Software Developers: Skilled software developers are needed to create the infrastructure and applications that interact with AI models and integrate them into existing systems.
- Project Managers and Business Analysts: These professionals oversee project planning and execution and ensure alignment with business goals, contributing to project management costs.
Hardware and Software Costs
As mentioned previously, hardware and software costs (infrastructure) can significantly contribute to the cost of developing and maintaining AI applications. These costs include:
- Computing Resources: Powerful hardware, such as GPUs or specialized AI accelerators, is essential for training and running AI models. The cost of acquiring and maintaining this hardware can be substantial, especially as the demand for this hardware increases.
- Software Licenses and Subscriptions: AI development and deployment often require licenses for specialized software frameworks, libraries, and tools. Additionally, ongoing subscriptions to cloud services or AI platforms may be necessary for your AI system.
Data Costs
AI needs data, and a lot of it. Acquiring and storing this data can cost a lot of money, especially for real-time AI systems that require breakneck read/write speeds and more specialized database technologies, such as graph or vector databases. Not to mention the ETL costs of getting data from one place to another in the correct format. Here are the areas to consider when budgeting this out:
- Data Acquisition: Gathering and cleaning relevant data can be costly, especially when acquiring external datasets or manual annotation.
- Data Storage: Storing and managing large volumes of data for AI training and inference requires cutting-edge and potentially expensive storage solutions.
Operational Costs
Once deployed, AI systems will require ongoing operational budgets to ensure the applications meet user demands. This may impact the areas we already touched on, such as updating a database to the latest version or deploying a monitoring solution on its own infrastructure. These are the areas to take into consideration when budgeting for the current and future operational costs:
- Maintenance and Updates: AI systems need ongoing updates to ensure optimal performance, security, and compliance with evolving regulations.
- Monitoring and Support: Real-time monitoring and technical support may be required to address any issues that arise during AI system operation.
By understanding these cost components, organizations can create a comprehensive budget for their AI initiatives, appropriately allocate resources, and make informed decisions throughout the development and deployment to keep the project on budget.
AI Cost Analysis Example
Putting this into practice, let’s create a simple AI cost analysis for a fictitious Text-to-Speech (TTS) API. Remember, this is for demonstration purposes only, so the numbers I’m using may vary depending on what part of the world you are building this in and the scope of what you are creating. Here’s what a simple AI cost analysis would look like for such an API:
Project Name: AI-Powered Text-to-Voice API
Goal: To develop and deploy a high-quality, customizable Text-to-Voice API that converts written text into natural-sounding speech for various applications.
Timeline: 9 months
Cost Breakdown:
- Personnel Costs:
- Machine Learning Engineer (2 FTEs): $220,000 annual salary (combined)
- Software Engineer (2 FTEs): $180,000 annual salary (combined)
- Linguistics Expert (0.5 FTE): $50,000 annual salary
- Project Manager (0.5 FTE): $60,000 annual salary
- Total Personnel Costs: $460,000
- Hardware and Software Costs:
- High-Performance Servers (2): $20,000 (one-time cost)
- GPUs for Model Training (4): $16,000 (one-time cost)
- Deep Learning Frameworks (TensorFlow, PyTorch, etc.): $5,000 annual subscription
- Cloud Computing Resources (AWS/Azure): $20,000 estimated annual cost
- API Development and Management Tools: $8,000 annual subscription
- Total Hardware and Software Costs: $69,000 (first year)
- Data Costs:
- Voice Dataset Acquisition and Licensing: $30,000 (one-time cost)
- Text Corpus for Training (Public Domain/Scraped): $0
- Data Cleaning and Preprocessing: $8,000 (estimated)
- Data Storage (Cloud-based): $4,000 annual cost
- Total Data Costs: $42,000 (first year)
- Operational Costs:
- Ongoing Model Refinement and Updates: $15,000 estimated annual cost
- API Monitoring, Scaling, and Security: $12,000 estimated annual cost
- Customer Support and Documentation: $6,000 estimated annual cost
- Total Operational Costs: $33,000 (annual)
Total Estimated Project Costs:
- Year 1: $574,000
- Year 2 Onwards: $33,000 (annual maintenance and operational costs)
Additional Considerations:
- This cost breakdown is simplified and may not capture all potential expenses.
- Voice dataset acquisition and licensing can be a significant variable cost depending on the quality, language coverage, and desired accents.
- The costs of cloud computing resources can fluctuate based on usage and scaling needs.
- Consider potential revenue streams through API usage fees or subscription models.
Expected Benefits (ROI):
- Potential for high demand for TTS APIs across various industries (e.g., accessibility, education, entertainment).
- Recurring revenue from API usage or subscriptions.
- Competitive advantage by offering high-quality, customizable TTS voices.
- Reduced development costs for businesses that would otherwise need to build their own TTS solutions.
For ROI, we would likely want to dig in a bit further with revenue projections, but this is more involved than what we will get into in this blog. That being said, by carefully analyzing costs and potential revenue streams, organizations can make informed decisions about developing applications and APIs, such as an AI-powered Text-to-Voice API, and position themselves for success in the current AI goldrush.
Using Moesif for AI Cost Analysis
Managing and optimizing AI costs can be complex, but tools like Moesif offer valuable insights that can help determine your AI solution’s actual cost and revenue. Moesif is an API analytics platform that provides insights into AI system usage, performance, and associated costs. Here’s how Moesif can assist in AI cost analysis:
- Detailed Usage Tracking: Moesif allows you to track API calls made to your AI systems, providing granular data on usage patterns, peak hours, and resource consumption (if it’s included as part of the API request or response). This data helps identify areas where optimization is possible, potentially helping to bring down AI API costs.
- Cost Allocation: Moesif enables accurate cost allocation by associating API calls with specific users and companies. This transparency helps identify cost drivers and make informed resource allocation and feature development decisions.
- Anomaly Detection: Moesif can detect unusual spikes in API usage or factors leading to unexpected cost increases, such as an increase in outgoing API calls to services you are paying for. By alerting you to potential issues or inefficiencies in your AI systems, Moesif can help teams remedy issues in real-time.
- Performance Monitoring: By monitoring API response times and error rates, Moesif helps ensure that your AI systems perform optimally and deliver value to users.
- Customizable Dashboards: Moesif offers customizable dashboards to visualize AI usage and cost data in a way that aligns with your organization’s or project’s specific needs and goals.
- Monetization Capabilities: AI platforms need to drive revenue. With Moesif, organizations can enable usage-based monetization of their AI apps with support for pre-paid and post-paid billing schemes. AI usage can be aggregated at a fine-grained level and then passed to popular billing providers such as Stripe, Chargebee, and many more.
By leveraging Moesif’s analytics and monetization capabilities, organizations can gain a deeper understanding of their AI costs, identify areas for optimization, make data-driven decisions to improve the efficiency and ROI of their AI investments, and drive revenue.
Conclusion
Building and implementing AI systems involves complex costs, ranging from data acquisition and infrastructure to model development and ongoing maintenance. Understanding these cost factors and employing effective strategies to optimize expenses is crucial for organizations aiming to harness the power of AI while ensuring a positive return on investment.
Organizations can reduce costs and accelerate time-to-market by carefully planning projects, starting with minimum viable products, leveraging pre-trained models, and embracing iterative development. Tools like Moesif can provide valuable insights into AI usage, performance, and costs, enabling data-driven decisions and continuous improvement.
Ready to take control of your AI costs and maximize the value and revenue of your AI investments? Sign up today to discover how Moesif can empower your organization as a key part of your AI cost analysis and optimization efforts. Explore our features and see how we can help you unlock AI’s full potential while keeping costs in check.