Maximizing Business Impact: Best Practices of AI Product Analytics
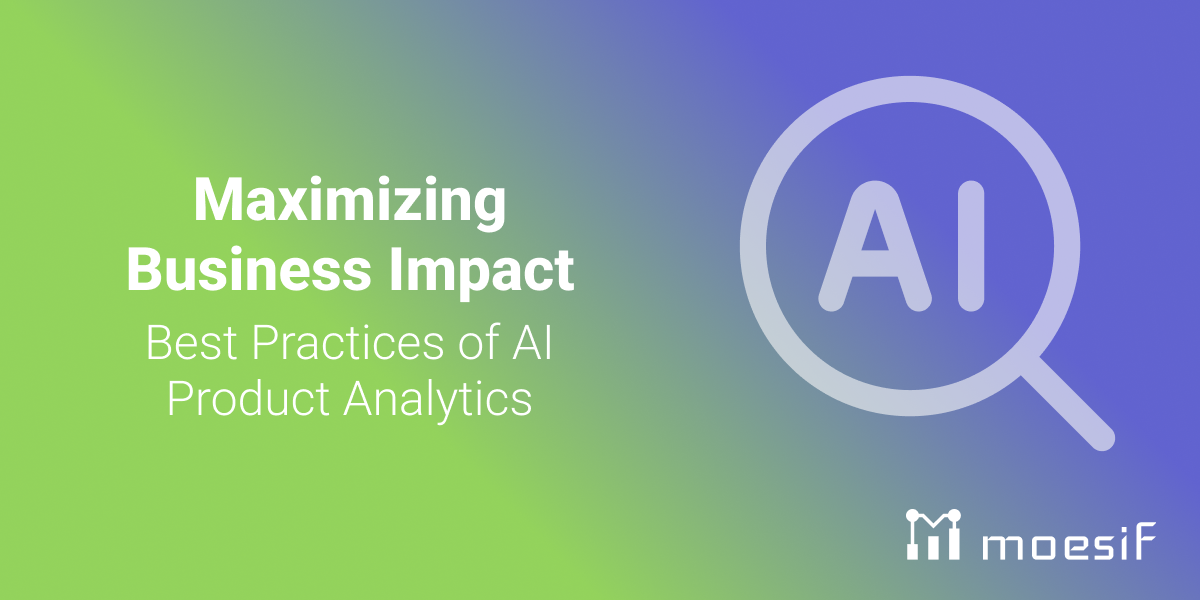
According to Gartner, 87% of organizations are classified as having low business intelligence and analytics maturity, meaning they struggle to extract value from their data.
This alarming statistic highlights a common struggle—turning raw data into actionable insights. Product teams often find themselves overwhelmed by the sheer volume of information they collect. Extracting meaningful patterns, deciphering user behavior, and predicting market trends from this sea of customer data can seem daunting.
AI product analytics offers a powerful solution, transforming complex datasets into clear, actionable insights. However, to truly harness its potential, teams must go beyond mere implementation and adopt best practices that align with their unique business goals.
In this article, we’ll guide you through the most effective strategies to bridge the gap between data and decision-making. Whether you’re a beginner developer trying to understand the basics or a seasoned data professional looking to refine your approach, this article provides valuable insights to elevate your product strategy.
Table of Contents
- What is AI Product Analytics?
- Understanding AI in Product Analysis
- Key Elements of AI Analytics
- The AI-Driven Product Analysis Process
- Benefits of AI-Enhanced Product Analysis
- Use Cases for AI Product Analytics
- Implementing AI Product Analytics
- Best Practices for AI Product Analytics
- Future Trends in AI-Enhanced Product Analysis
- Measuring the Impact of AI Product Analytics
- Conclusion
What is AI Product Analytics?
At its core, AI product analytics leverages the power of artificial intelligence to analyze and interpret data from product interactions. This data encompasses a wide range of information, including how users interact with your product, their purchasing behavior, and feedback. AI product analytics transforms this raw data into actionable insights, revealing patterns, trends, and opportunities that traditional methods might miss.
Unlike traditional analytics, AI product analytics employs machine learning algorithms and statistical models. These tools can handle massive datasets, uncover complex relationships, and generate predictions with remarkable accuracy. As a result, businesses gain a deeper understanding of their users, products, and market, allowing them to make informed decisions that drive growth.
Natural language processing further enhances AI product analytics by enabling users to interact with data through intuitive, conversational queries. It allows platforms to interpret questions in plain language and deliver relevant insights. This way, data analysis becomes more accessible to non-technical team members and improves overall decision-making capabilities.
Through proper use, AI-powered product analytics can bring significant benefits. It empowers product teams to pinpoint areas for improvement, personalize user experiences, optimize pricing strategies, and even predict future trends. Businesses can move from reactive decision-making to proactive strategies. This gives businesses a competitive edge in today’s fast-paced digital landscape.
AI product analytics also excels at processing both structured data and unstructured data. It analyzes quantitative metrics like sales figures and qualitative data like user reviews and social media sentiment. This comprehensive view provides a nuanced understanding of user behavior and market dynamics.
Understanding AI in Product Analysis
AI transforms how businesses approach product analysis. AI algorithms interpret data to derive insights that aid in decision-making processes. Predictive analysis can forecast future trends and behaviors through the analysis of historical and real-time data. It automates complex workflows to uncovers hidden patterns, allowing teams to make faster and more data-driven decisions. AI product analytics tools act as tireless data analysts. A company that has adopted such tools can process and analyze data in vast amounts to extract meaningful insights.
The Role of AI in Modern Product Analysis
AI helps businesses maintain deeper insights into user needs and behaviors. Traditional analytics might explain what users are doing, but AI reveals why they do it. This comprehensive understanding allows for the creation of products and experiences that resonate with your target audience.
Staying ahead in the competitive landscape means driving your business toward sustained growth. AI product analytics identifies emerging trends before they become mainstream. It allows you adapt your product strategy proactively and keep your offerings relevant and appealing to users.
A significant challenge in product analysis stems from handling large volumes of data. AI excels at processing massive datasets. It can draw data-driven insights from historical data, real-time interactions, and external market trends quickly. This speed provides a competitive advantage that manual analysis can’t match.
The power of AI in product analysis lies in real-time insights. You no longer have to wait weeks to analyze data. AI can help you track user behavior, monitor product performance, and identify issues as they happen. You can easily afford to make quick adjustments that enhance user experience and drive business growth.
Key Elements of AI Analytics
AI analytics relies on several key elements to transform raw data into meaningful insights. The process starts with data collection and processing, which forms the foundation for everything that follows.
Data Collection and Processing
Effective AI analytics depends on thorough data collection that involves gathering relevant and accurate data from multiple sources. These sources include user interactions, website analytics, customer feedback, social media sentiment, and external market data. A diverse set of data streams offer a comprehensive view of your product and its environment.
After collection, the raw data undergoes processing to prepare it for analysis. This stage includes cleaning the data, transforming it into a usable format, and correcting inconsistencies or errors. Effective data processing ensures that AI algorithms access high-quality, reliable information, leading to accurate and meaningful insights.
AI algorithms excel in analyzing both structured data, like sales figures and demographics, and unstructured data, like text reviews and social media posts. This capability allows businesses to extract insights from a wide range of sources, offering a more holistic understanding of products and users.
Analyzing unstructured data sets AI analytics apart from traditional methods. While conventional techniques struggle with text, images, and audio, AI algorithms can extract valuable insights from these formats. This ability helps you understand user sentiment and identify patterns that reveal emerging trends.
Effective data collection and processing lay the groundwork for AI to reveal valuable information.
The AI-Driven Product Analysis Process
AI-driven product analysis involves several interconnected steps that together provide a comprehensive understanding of your product, users, and market.
Analyzing Historical Data
Historical data offers valuable insights into past user behavior, product performance, and market trends. AI algorithms interpret data to identify patterns and correlations that might not seem obvious. Understanding the past helps you make informed decisions for the future.
Real-Time Analysis
Real-time analysis is a powerful feature of AI product analytics. As users interact with your product, AI tracks their behavior, identifies potential issues, and predicts future actions. This enables proactive adjustments to improve user experience and reduce churn.
Predictive Modeling
AI product analytics not only reviews past events but also predicts future outcomes. By analyzing historical data and current trends, AI algorithms interpret data to generate forecasts on user behavior, product adoption, and market shifts. This foresight helps you anticipate challenges and seize opportunities ahead of your competition.
User Segmentation
AI product analytics enables the segmentation of your user base into distinct groups based on behavior, preferences, and demographics. This segmentation supports targeted marketing, personalized user experiences, and a product roadmap tailored to different user needs.
A/B Testing and Experimentation
AI enhances A/B testing and experimentation by analyzing variations and identifying the changes that improve engagement, conversions, and overall success. This evidence-based approach grounds product optimization decisions in concrete data.
Benefits of AI-Enhanced Product Analysis
AI-enhanced product analysis offers significant advantages, transforming how businesses understand their products and users.
- AI algorithms analyze large datasets quickly and precisely, reducing the risk of human error and bias. By automating repetitive tasks, AI allows your team to focus more on strategic initiatives.
- AI product analytics uncovers hidden patterns and motivations behind user behavior. It goes beyond surface-level metrics to interpret data, revealing deeper insights that allow you to create personalized experiences, targeted marketing, and products that resonate with your audience.
- AI-powered insights help you anticipate user needs and market trends. This include identifying churn risks or predicting what features most appeal to your users.
- AI product analytics can optimize product development, marketing, and customer support. This significantly boosts your return on investment (ROI). You can allocate resources more effectively, target the right users, and drive revenue and growth.
- AI product analytics provides a crucial edge in a competitive landscape. By leveraging AI for deeper insights and smarter decisions, you can outperform rivals, attract and retain more users, and position your brand as an industry leader.
Use Cases for AI Product Analytics
The applications of AI product analytics span across the entire product lifecycle, from development and design to marketing and customer support. Let’s explore some of the most impactful use cases.
Product Development and Design
AI product analytics guides development and design decisions by analyzing user feedback and usage patterns. You can identify areas for improvement, discover unmet needs, and prioritize features that matter most to users. This ensures your product evolves in line with user expectations and market demands.
Marketing and Sales
AI product analytics enhances marketing and sales efforts by understanding user behavior and preferences. This allows you to conduct targeted campaigns for specific audience segments. Moreover, you can optimize pricing strategies and identify cross-selling and upselling opportunities. Personalizing the customer journey like this increases conversions and revenue.
Customer Support and Success
AI product analytics improves customer support and success by analyzing customer behavior, interactions, and feedback. It identifies common pain points so you can address them proactively, boosting user satisfaction and reducing churn. AI also personalizes support interactions, offering solutions tailored to each customer.
Fraud Detection and Prevention
AI product analytics plays a crucial role in detecting and preventing fraud. It can identify unusual patterns and anomalies in user behavior. Having a proactive and automatic threat detection system protects your business and your users from potential threats in real time.
Competitive Analysis
AI product analytics helps you stay ahead of competitors by tracking their performance and analyzing their strategies. It identifies strengths and weaknesses and benchmarks your product against industry standards. Consequently, you can make informed decisions that differentiate your product and capture market share.
Implementing AI Product Analytics
Implementing AI product analytics requires more than selecting the right tools. If you want to succeed, you must also invest heavily in careful planning, execution, and ongoing optimization. Here we briefly discuss some key areas that we recommend you consider when implementing AI-powered product and data analytics.
Data Quality and Security
Ensure data quality in AI product analytics by collecting relevant, complete, and accurate data. Validate and clean data regularly to maintain integrity and avoid skewed results.
Protect sensitive user information by implementing encryption, access controls, and conducting regular audits. Strong security measures build and maintain user trust.
Choosing the Right Solution
Select the right AI product analytics solution by evaluating vendors and platforms on ease of use, scalability, integration, and support. Choose a solution that aligns with your business needs and technical requirements.
Collaborative Implementation
Successful implementation requires collaboration among data scientists, product managers, engineers, and stakeholders. Promote open communication and cross-functional collaboration. This makes sure that everyone understands and utilizes insights for product improvement.
Ongoing Optimization
Monitor and evaluate AI models continuously. Refine data collection and processing strategies, and adapt as business and user needs evolve. Embrace a culture of learning and experimentation to maximize the value of AI product analytics.
Best Practices for AI Product Analytics
To fully harness the power of AI product analytics, adopt best practices that align with your business objectives and ensure effectiveness.
Aligning AI Analytics with Business Goals
Start by clearly defining your business goals. Determine whether you aim to increase user engagement, boost conversions, or improve customer retention. With clear objectives in mind, tailor your AI analytics strategy to support these goals.
Choosing the Right Metrics
Select metrics that directly measure progress toward your business goals. Key performance indicators (KPIs) should align with your objectives, such as user retention rate, conversion rate, average revenue per user, or customer satisfaction scores. These metrics guide the evaluation of your AI product analytics initiatives.
Building a Data-Driven Culture
Foster a data-driven culture to maximize the benefits of AI product analytics. Encourage your team to make decisions based on data at all levels. Provide training and resources to help them interpret AI-generated insights and apply these insights to their work.
Investing in Talent and Technology
Successful AI product analytics requires skilled talent and the right technology. Invest in hiring or training data scientists and analysts with AI expertise. Choose AI product analytics tools that are user-friendly, scalable, and compatible with your existing technology stack.
Continuous Improvement and Iteration
AI product analytics requires ongoing learning and improvement. Regularly monitor and evaluate the performance of your AI models. Continuously refine your data collection and processing strategies, and adapt your approach as business and user needs change.
Ensuring Data Privacy and Compliance
AI product analytics often involves handling sensitive user data. Therefore, make sure that your data collection, storage, and processing practices comply with relevant data privacy regulations, such as GDPR or CCPA. Regularly review your data practices to maintain compliance and protect user privacy. This not only safeguards your business legally but also builds trust with your users.
Future Trends in AI-Enhanced Product Analysis
AI product analytics continues to evolve through technological advancements and changing business needs. Let’s briefly look at some of the trends that can impact its trajectory in the days to come.
- AI product analytics will become even more powerful as algorithms grow more sophisticated. These advancements will unlock deeper insights and predictive capabilities, integrating AI into every phase of the product lifecycle.
- Real-time personalization represents a significant future trend. AI will allow businesses to tailor product experiences instantly, based on user behavior, preferences, and context. This immediate personalization will boost user engagement and satisfaction.
- Predictive analytics will take center stage in the future of AI product analysis. AI will help businesses anticipate user needs and market trends, enabling proactive decisions that drive growth and innovation. This might include predicting churn or identifying new product opportunities.
- As AI models become more complex, the demand for explainable AI will grow. Businesses will need transparency in how AI models generate insights. This transparency will build trust and help teams understand the factors influencing user behavior and product performance.
- The importance of ethical AI in product analytics will continue to rise. Ensuring AI models are fair, unbiased, and respectful of user privacy will be crucial factors. Businesses must implement responsible AI practices to benefit both the company and its users.
Measuring the Impact of AI Product Analytics
So you’ve incorporated AI into your product analysis or thinking about doing so. You likely want to understand how much value it adds to your business. This may likely decide the next steps you want to take.
The primary goal of AI product analytics is to drive positive business outcomes. These outcomes may include increased revenue, improved user satisfaction, reduced churn, or enhanced operational efficiency. Track key performance indicators (KPIs) aligned with your business goals to quantify the impact of your AI initiatives.
Choosing relevant KPIs is crucial for effective measurement. Select metrics that directly reflect the impact of AI product analytics on your business. For instance, if you want to increase user engagement, track metrics like time spent in-app, feature adoption rates, and user retention.
Before implementing AI product analytics, establish a baseline for your chosen KPIs. This baseline allows you to compare performance before and after implementation, providing a clear picture of AI’s impact on your business.
Monitor your KPIs regularly and generate reports that highlight the impact of AI product analytics. Share these reports with key stakeholders to demonstrate the value of your initiatives and secure ongoing support.
Use insights from your measurements to refine strategies and optimize your approach. Identify areas where AI delivers the most value and areas needing improvement. Continuously iterating and adapting ensures AI product analytics remains a strong driver of business success.
Conclusion
With the democratization of AI, AI tools and services have become more accessible. We have seen traditional product analytics tools add artificial intelligence support over the years as well, for example, Mixpanel. Like many other aspects of modern business landscape, AI will likely dominate product analysis as well. Small to large companies alike will lean heavily into bespoke AI solutions when it comes to product-related data analysis in the coming years.
AI adoption paints only half of the picture. The rest depends on choosing the right tools to monitor, analyze, and monetize your AI product. And we’ve built Moesif from the ground up as a comprehensive platform that can maximize the value of your product’s entire lifecycle.
Moesif offers a comprehensive suite of powerful analytics and monitoring tools and robust monetization features so you can confidently grow your AI product.
Sign up today for a free trial today to try it yourself, no credit card required.