Enhancing AI Customer Experience: A Practical Guide
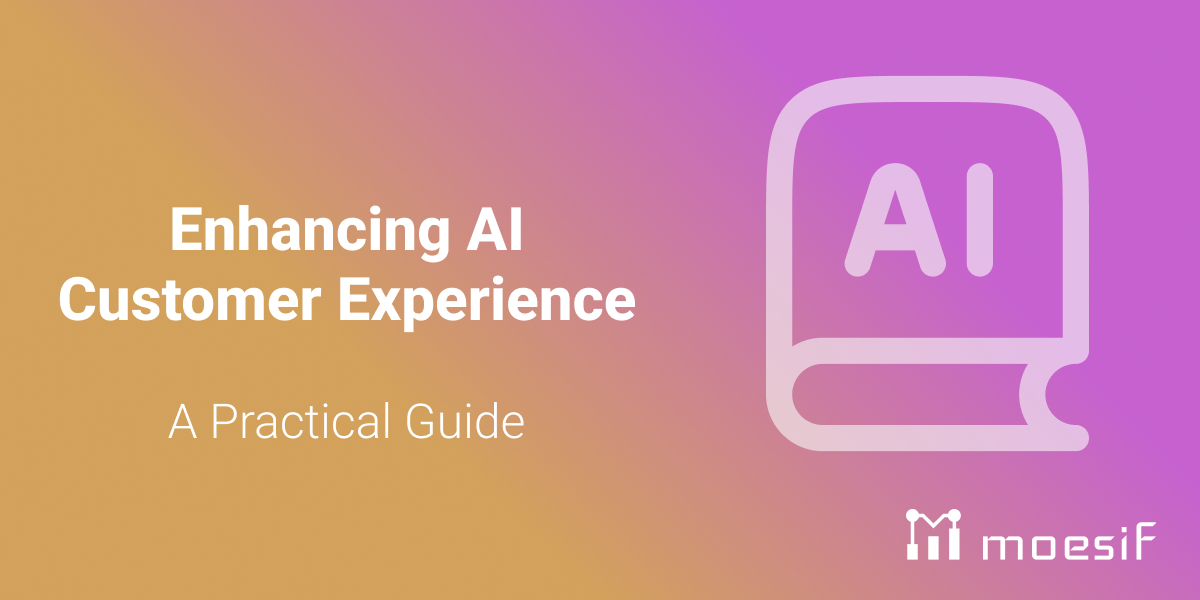
Organizations are harnessing the power of AI to revolutionize products and services across industries. But AI-powered solutions have been getting more sophisticated. We need to redesign and amend our approach to understanding how customers experience these solutions.
Unlike traditional products, AI solutions are dynamic, continuously learning and adapting. Traditional metrics may fall short of capturing the nuances of how users interact with AI. We need better ways to understand and anticipate customer needs.
This article delves into why AI customer experience (CX) is different, explores the key metrics and customer data you should be tracking, and outlines strategies for building a comprehensive CX program.
Table of Contents
- Why AI Customer Experience is Different (and Why it Matters)
- Why It Matters
- Key Metrics for Tracking AI Customer Experience
- Building a Comprehensive AI CX Strategy
- Conclusion
Why AI Customer Experience is Different (and Why it Matters)
The rise of artificial intelligence has ushered in a new era of customer experiences. AI products demand a fresh perspective and approach. Let’s discuss this in more detail.
The Ever-Evolving Nature of AI
Unlike static products, AI solutions are dynamic. They constantly learn and adapt from new data inputs and interactions. Some solutions can analyze customer behavior to change over time. This perpetual evolution means that the customer experience with AI can shift and improve—or sometimes regress—over time. Therefore, you must monitor customer experience with continuous attention and adjustment. Companies must invest in tools and practices that allow them to track these changes effectively, ensuring that the AI systems continue to meet customer needs and expectations.
The dynamic nature of AI means that the experience doesn’t maintain uniformity across users or time. Different customers may have varying experiences based on their interactions, contexts, or even device differences. This variability requires businesses to optimize every interaction for the individual user. To implement this personalized approach, you need advanced analytics and feedback mechanisms to capture and respond to customer sentiments in real-time.
The Black Box Problem
AI decision-making processes are often complex and opaque, sometimes even to the developers who created them. This lack of transparency can lead to mistrust or confusion among users. To address this challenge, AI customer experience must prioritize explainability and transparency so that users understand the how and why behind the decisions. Clear, understandable explanations can build trust and confidence, helping users feel more comfortable with AI interactions.
Customers understanding the rationale behind AI decisions leads to more positive and constructive engagement. Therefore, try developing user-friendly interfaces and communication strategies that demystify AI processes. By focusing on explainability, companies can create a more trustworthy relationship with their customers.
The Impact of Bias
AI models have as much value as the data you train them on. Unfortunately, this data can sometimes carry biases that lead to unfair or discriminatory outcomes. This can result in a compromised customer experience, particularly for marginalized or underrepresented groups. Companies must actively monitor their AI systems for bias and implement strategies to so that their AI solutions are fair and equitable for all users.
Addressing bias in AI not only poses technical challenges but also an ethical imperative. Organizations must commit to ethical AI practices. They must recognize the fact that without unbiased AI, you cannot build trust and maintain a positive reputation.
By prioritizing fairness and inclusivity in their AI customer experience strategies, companies can foster a more equitable environment where all users feel valued and respected. This commitment to ethical AI can also set a company apart in the marketplace, enhancing brand loyalty and customer satisfaction.
The Emotional Connection
As AI becomes more sophisticated, it can evoke a wide range of emotions in users, from delight to frustration, and even fear. You can craft a positive customer experience by understanding the emotional impact of AI interactions. AI customer experience strategies must consider these emotional dynamics, striving to build trust and rapport with users. This involves designing AI systems that have empathy and responsiveness, capable of recognizing and adapting to users’ emotional states.
Creating an emotional connection with users goes beyond functionality. It requires a deep understanding of human behavior and psychology. In that regard, companies can leverage emotional intelligence in AI design and natural language processing (NLP). This allows them to create personalized service and more meaningful interactions that resonate with users. It will also assist and compliment customer service agents.
The High Stakes of Error
In sensitive domains like healthcare or finance, AI errors can have significant consequences. Mistakes can lead to severe financial or personal repercussions for users.
Therefore, we must ensure the reliability and accuracy of AI systems in these domains. AI customer experience must prioritize error detection and correction, implementing robust protocols to mitigate the impact of any mistakes. A comprehensive approach to quality assurance, involving rigorous testing and validation processes, can ensure that AI solutions perform as intended.
In addition to technical safeguards, companies must also develop effective communication strategies for handling errors. You want to maintain transparency and accountability when addressing AI mistakes. Users feel assured that you are considering their issues seriously and working on promptly resolving them.
By proactively addressing errors and learning from them, organizations can enhance trust and confidence in their AI systems. This ultimately improves the overall customer experience and in the long run ensures the success of AI-driven initiatives.
Why It Matters
AI customer experience (CX) influences how companies interact with their customers and leverage AI technology to drive business success. AI CX builds trust—one of the key reasons it matters.
In this era, AI’s presence dominates our everyday lives. Therefore, transparent and explainable AI systems foster trust among users. Customer understanding of how AI makes decisions results in long-term adoption and engagement. Trust, the foundation of any successful relationship, gives users confidence and security in AI-driven products and services.
Mitigating risk is another critical aspect. Proactively addressing potential biases and errors within AI systems can safeguard brand reputation and avoid negative consequences for users. This involves implementing rigorous testing and monitoring processes to identify and rectify issues before they escalate. A proactive approach to risk management can prevent incidents that can damage customer trust and loyalty. Moreover, demonstrating a commitment to ethical AI practices enhances a company’s reputation as a responsible and trustworthy brand.
AI CX also drives innovation. The data generated through AI customer interactions provides valuable insights into user behavior and preferences. By analyzing this data, companies can identify opportunities for developing new and improved AI features that better meet customer needs. You can use predictive analytics to analyze customer sentiment and behavior to prevent churn and facilitate meaningful interactions. This iterative approach ensures that AI solutions remain relevant and effective, continuously enhancing the customer experience.
Excelling at AI CX also gives you a competitive advantage. Companies that master the nuances of AI customer experience can attract and retain customers better in an increasingly AI-driven marketplace.
As AI continues to reshape industries and consumer expectations, businesses that prioritize exceptional AI CX will differentiate themselves from competitors. This involves not only delivering superior products and services but also providing seamless and personalized experiences that resonate with users.
Key Metrics for Tracking AI Customer Experience
To effectively measure and improve the AI customer experience, you want a multi-faceted approach that combines quantitative and qualitative data. It helps capture a comprehensive view of how users interact with AI solutions and provides insights into areas that need enhancement. By focusing on the right metrics, organizations can gain valuable insights into user behavior and satisfaction. Here are the key metrics you should be tracking:
Usage Metrics
Time Spent Using the AI
This metric provides insight into how engaging and valuable users find your AI solution. Longer interaction times often indicate a positive experience. It suggests that users are finding the tool beneficial and engaging. Conversely, short interaction times might suggest confusion, frustration, or a lack of perceived value, highlighting areas for improvement. By analyzing this data, companies can identify patterns and make informed decisions to enhance user engagement and satisfaction.
Features Most Utilized
Understanding the most popular features can help prioritize development efforts and optimize the user interface. By identifying high-usage features, organizations can focus on refining and expanding these areas to maximize user satisfaction. Additionally, this metric can reveal underutilized features that may need better visibility or improvement.
Frequency of Interactions
Frequent interactions suggest that your AI is meeting a genuine need for your users and has become an integral part of their workflow. This metric indicates that users are consistently finding value in the AI solution, leading to habitual use. Analyzing interaction frequency and customer inquiries can help identify trends and patterns in user behavior, providing insights into how the AI solution fits into users’ daily routines.
Performance Metrics
Measuring the performance of AI systems ensures that they meet user expectations and deliver value. Performance metrics focus on the technical capabilities of AI solutions, which directly impact user satisfaction and trust. By continuously monitoring these metrics, companies can make necessary improvements to maintain high standards of service. Here are the key performance metrics to consider:
Accuracy of AI Responses
Inaccurate responses can quickly erode trust and lead to user frustration. Users rely on AI systems to provide reliable information and solutions. Any deviation from this expectation can have significant consequences. Continuously monitoring and improving your AI’s accuracy maintains user confidence and satisfaction. This involves regular updates and training of AI models to ensure they remain aligned with user needs and current information.
Speed of Processing
In today’s fast-paced world, users expect quick responses from AI systems. The speed of processing directly affects user experience, as delays can lead to frustration and decreased engagement. To meet user expectations and keep them engaged with your solution, monitor processing times and optimize your algorithms for speed. A fast and efficient AI system can differentiate your product in a competitive market and enhance overall user satisfaction.
Task Completion Rates
This metric measures the effectiveness of your AI in helping users achieve their goals. High task completion rates signal a successful AI solution that effectively guides users through processes and assists them in reaching their desired outcomes. By tracking task completion rates, organizations can identify areas where users may struggle and make improvements to enhance the AI’s effectiveness. This focus on successful task completion reinforces the value of the AI solution and contributes to positive user experiences.
User Satisfaction Metrics
User satisfaction reflects how well the AI solution meets users’ needs and expectations. User satisfaction metrics provide organizations valuable insights into the strengths and weaknesses of their AI products. Here are the key metrics to track user satisfaction:
Surveys and Ratings
To gauge overall user satisfaction, regularly collect feedback through surveys and rating scales. These tools allow users to provide structured feedback on various aspects of the AI experience, such as usability, accuracy, and efficiency. By asking specific questions about different elements of the AI interaction, companies can identify areas that require improvement and areas that are performing well. This quantitative data provides a clear picture of user sentiment. Ultimately, it can guide strategic decisions towards better customer experience by enhancing customer engagement through interactions and data analytics.
Feedback Forms
Providing open-ended feedback forms allows users to express their thoughts and suggestions in detail, offering qualitative insights that structured surveys might miss. These forms give users the opportunity to share unique experiences, highlight specific pain points, and suggest improvements. By encouraging detailed feedback, organizations can gain a deeper understanding of user needs and preferences, enabling them to tailor their AI solutions more effectively. This direct line of communication with users fosters a sense of involvement and engagement, enhancing overall satisfaction.
Sentiment Analysis
Utilizing AI-powered sentiment analysis tools to analyze user comments and reviews can help identify areas of positive and negative sentiment. These tools can process large volumes of text data, extracting valuable insights into how users perceive the AI solution. By pinpointing common themes and emotions in user feedback, companies can quickly identify strengths to build upon and weaknesses to address. Sentiment analysis provides a comprehensive view of user attitudes and helps prioritize areas for improvement, ensuring that the AI product evolves in line with user expectations.
Behavioral Metrics
Behavioral metrics provide insights into how users interact with AI solutions, revealing patterns and tendencies that can inform design and development strategies. Understanding user behavior helps organizations optimize the user journey and enhance the overall experience. Here are key behavioral metrics to track:
Drop-Off Points
Analyzing where users abandon interactions with your AI can help identify areas for improvement in the user journey. Drop-off points indicate where users encounter obstacles, confusion, or frustration, prompting them to exit the process prematurely. By pinpointing these critical junctures, companies can investigate underlying causes and implement changes to streamline the experience, reduce friction, and encourage users to complete their interactions. Addressing drop-off points can improve retention and ensure users derive maximum value from the AI solution.
User Flow Analysis
Tracking how users navigate through your AI solution provides valuable insights into user behavior and preferences. AI should complement human interaction to optimize customer engagement. User flow analysis helps identify bottlenecks or areas where users might get stuck. This allows companies to enhance navigation and optimize pathways. This analysis also helps identify the most accessed and the most overlooked features. As a result, you get a basis for targeted enhancements and prioritization.
Feature Adoption Rates
Adoption rates of new AI features and their usage frequencies can help you understand engagement and product relevance. High adoption rates suggest that features are meeting user needs and are effectively communicated. Low adoption rates may indicate a lack of awareness or perceived value. Feature adoption tracking allows organizations to assess the impact of new releases, refine their product roadmap, and prioritize features that resonate most with users. Understanding adoption trends also opens up data-driven decision making.
Error Analysis
Error analysis helps identify and rectify issues that can negatively impact user satisfaction and trust. By systematically analyzing errors, organizations can improve their AI systems. Here are the key components of effective error analysis:
Identifying and Categorizing Errors
Companies should implement robust error tracking mechanisms that can log errors in real-time and classify them based on their nature, frequency, and impact. By categorizing errors, companies can prioritize which issues to address first, focusing on those that have the most significant effect on user experience. This systematic approach allows organizations to pinpoint specific areas in their algorithms that require improvement.
Root Cause Analysis
Conducting root cause analysis involves digging deeper into the underlying causes of AI errors to identify patterns and trends. Rather than simply addressing surface-level problems, root cause analysis seeks to uncover systemic issues that may be contributing to recurring errors. By analyzing the data collected from error tracking, organizations can identify common factors or conditions that lead to failures. This comprehensive understanding enables companies to implement long-term solutions that prevent similar issues from arising in the future, enhancing the robustness and accuracy of their AI systems.
By tracking these key metrics, you’ll gain a comprehensive understanding of how users are experiencing your AI product. This data can then be used to inform product development decisions, enhance user satisfaction, and ultimately build a more successful AI solution.
Building a Comprehensive AI CX Strategy
Crafting a robust AI customer experience strategy requires a holistic approach that encompasses data collection, feedback loops, experimentation, and transparency. A well-rounded strategy ensures that AI solutions meet user needs effectively and adapt to changing expectations. Here’s how to create a winning AI CX strategy:
Data Collection: The Foundation of Your Strategy
Track Across All Touchpoints in the Customer Journey
Implement tracking mechanisms across all user interactions with your AI, including websites, apps, chatbots, voice assistants, and customer service interactions. Comprehensive tracking allows you to gather insights from every stage of the customer journey, helping you understand how users engage with your AI solutions in different contexts. This allows organizations to identify areas for improvement and ensure a seamless, consistent experience across all platforms and devices.
Capture Qualitative and Quantitative Data
To gain a complete picture of the user experience, gather both numerical data (for example, usage metrics, performance metrics) and qualitative data (for example, user feedback, sentiment analysis). Quantitative data provides insights into user behavior and system performance, while qualitative data offers deeper understanding into user perceptions and emotional responses. By combining these data types, companies can identify strengths and weaknesses in their AI solutions, making informed decisions to enhance customer satisfaction and engagement.
Leverage User Analytics Platforms
Utilize user analytics platforms like Mixpanel, Amplitude, and Google Analytics to collect, analyze, and visualize user data. These tools offer powerful features for tracking user interactions, measuring key performance indicators, and identifying trends. By leveraging analytics platforms, organizations can gain actionable insights into user behavior, helping them optimize their AI solutions and drive continuous improvement.
Integrate with CRM Systems
Connect your AI CX data with your customer relationship management (CRM) system to gain deeper insights into individual customer journeys and preferences. Sales teams can leverage this integrated data to gain insights into customer preferences and behaviors, allowing them to tailor their outreach and improve conversion rates. Integration with CRM systems allows companies to combine AI interaction data with customer profiles.
Feedback Loops: The Voice of Your Customers
Actively engage with users and incorporate their insights into your development processes. This way, you can create AI solutions that better meet user needs and expectations. Here are our tips to implement successful feedback loops:
Active Solicitation
Don’t wait for users to complain. Actively seek feedback through surveys, feedback forms, in-app prompts, and social media. By reaching out proactively, you can gather a wide range of opinions and insights, helping you understand both positive aspects and areas needing improvement. This proactive approach demonstrates to users that you value their opinions and have the commitment to deliver a superior experience.
Real-time Feedback
Incorporate feedback mechanisms that allow users to provide feedback in the moment, during their interactions with your AI. Real-time feedback tools, such as in-app surveys or feedback buttons, capture users’ thoughts and feelings as they occur. This provides you immediate insights into their experiences. Such a timely information can highlight issues or successes as they happen, allowing for quicker adjustments and improvements.
Incentivize Participation
Offer incentives like discounts or early access to new features to encourage users to share their feedback. Incentives can boost participation rates and ensure you gather a diverse range of perspectives. By rewarding users for their input, you achieve two things:
- Increase the quantity of feedback.
- Demonstrate appreciation for their time and effort.
In the end, you foster a positive relationship between your brand and its customers.
Act on Feedback
Analyze user feedback regularly and use it to inform product development decisions, prioritize bug fixes, and refine your AI models. Close the loop by showing users that their feedback leads to tangible improvements. By communicating updates and changes based on user input, you reinforce the value of their contributions. Moreover, you further solidify their trust in your commitment to continuous improvement.
Experimentation: The Path to Optimization
Experimentation is a critical component of developing and refining AI solutions, allowing companies to test hypotheses, evaluate changes, and optimize user experiences. By systematically experimenting with different aspects of AI products, organizations can make informed decisions that enhance performance and user satisfaction. Here’s how to implement effective experimentation:
A/B Testing
Conduct A/B tests to compare different versions of your AI models, features, or user interfaces. A/B testing involves presenting two or more variations to different user groups and analyzing which version performs better. This helps you identify the changes taht resonate best with users so that you have a clear evidence of what works and what doesn’t. A/B testing is a straightforward and effective method for optimizing specific elements of the AI customer experience.
Multivariate Testing
For more complex scenarios, use multivariate testing to evaluate multiple variables simultaneously and identify the optimal combination. A/B testing focuses on one variable at a time. On the other hand, multivariate testing examines how different variables interact with each other. It helps you understand the combined effects of changes and finding the best configuration for enhancing user experience. By testing various combinations, companies can uncover insights that simpler testing methods might not reveal.
Data-Driven Decision Making
Base your product decisions on the results of your experiments, not just gut feelings or assumptions. Data-driven decision-making ensures that your choices are supported by empirical evidence, reducing the risk of costly mistakes and improving the likelihood of success. By leveraging the insights gained from testing, organizations can prioritize changes that have a proven impact on user satisfaction and business outcomes. This approach fosters a culture of continuous improvement, where data informs every stage of the product development process.
Transparency: Building Trust with Users
Transparency builds trust and fosters positive relationships with users in the context of AI. By being open about how AI systems function and you handle user data, organizations can alleviate concerns and encourage greater acceptance and engagement with their AI solutions. Let’s discuss!
Explainable AI (XAI)
Implement XAI techniques to help users understand how your AI makes decisions. This can involve providing explanations for recommendations, predictions, or actions the AI takes. By offering clear, understandable insights into AI processes, users can gain confidence in the system’s reliability and fairness. Explainable AI not only builds trust but also empowers users to make informed decisions based on the AI’s outputs.
Transparent Data Practices
Clearly communicate how you collect, store, and use user data to improve your AI. Address any privacy concerns upfront and give users control over their data. Without transparency in data practices, you cannot build trust, especially as users become more aware of data privacy issues. Provide users with clear information about data handling policies and offer options for data control and consent. These steps reassure users that you’re using their information responsibly and ethically.
Open Communication
Maintain open channels of communication with users to address any questions or concerns they may have about your AI. Regular updates, user forums, and responsive customer support can all contribute to a more transparent relationship. By engaging users in dialogue and being receptive to their feedback, organizations can build stronger, more trusting relationships.
By incorporating these key elements into your AI CX strategy, you can create a feedback loop that continuously drives improvement and innovation. Stay adaptable, listen to your users, and be willing to iterate and evolve your approach over time.
Conclusion
Artificial intelligence has an ever-evolving environment. Therefore, tracking customer experience has become a strategic imperative. The companies that excel at AI customer experience will thrive in the AI-driven future. Don’t just build AI products; build AI products that your customers love. And for that, you need to prioritize CX tracking and focus on the user experience.
In a future article, we’ll explore some of the best tools and technologies that can help you collect, analyze, and act on AI customer experience data. But if you want to significantly set yourself apart in your AI CX strategy, consider adding Moesif to your stack of tools.
Moesif offers a comprehensive suite of powerful analytics and monitoring tools and robust monetization features. Moesif gives you the best of both worlds: a customer-centric analytics platform for first-class AI CX, and native monetization features to grow your AI product,
So sign up today for a free trial to try it yourself, no credit card required.